Engineering AI:
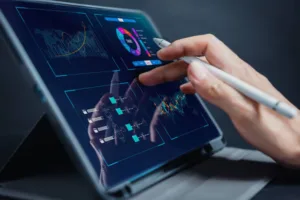
The realm of scientific inquiry is a complicated ecology in which ideas thrive, compete, and eventually affect the trajectory of human progress. But how can we accurately assess the impact of individual research endeavours in this huge landscape?
Historically, impact factors (IFs) have dominated, acting as a numerical representation of a journal’s prestige and, by extension, the “value” of the research it publishes. However, this one-size-fits-all approach is increasingly viewed as inadequate, failing to convey the complex character of scientific influence and potentially impeding innovation.
Due to these limitations, the exciting world of engineering AI research comes into play, where strong tools and fresh methodologies are changing the way we evaluate research effects. Researchers are going deeper than ever before, using artificial intelligence and machine learning to find hidden indicators and reveal a fuller picture of scientific influence.
Beyond the Journal: Unveiling the Spectrum of Impact Factors
One of the primary drawbacks of classic Impact Factors is their exclusive focus on journal prestige. While influential journals play an important role in research dissemination, they are merely one pathway of effect. Engineering AI research challenges this restrictive approach by investigating alternate sources of influence.
For example, academics are using citation analysis methods that go beyond mere counts to investigate the context and direction of citations. This enables a more detailed picture of how research is used, highlighting instances in which influential ideas are accepted and subsequently developed, even if they are not directly credited.
Furthermore, social media analytic techniques are being used to monitor online discussions and mentions of research, providing significant insights into its reach and participation beyond academia.
Read More: How Quantum-Powered AI is Revolutionising Industries
Exploring the Power of Network Analysis in Engineering AI
The interconnectedness of research provides another potential for AI-powered analysis. Network analysis tools enable researchers to visualise the relationships between various publications, authors, and organisations.
By examining these networks, AI systems may detect clusters of influential research, trace the flow of ideas, and even forecast the future significance of developing fields. This holistic approach provides a better grasp of the complex dynamics that drive scientific advancement, finding hidden jewels that would otherwise go overlooked.
Furthermore, AI-powered technologies may examine the textual content of research articles to extract essential themes, methodologies, and results. This data can then be used to identify new patterns, track the progress of research disciplines, and flag prospective breakthroughs that need to be explored.
What are the limitations of impact factors?
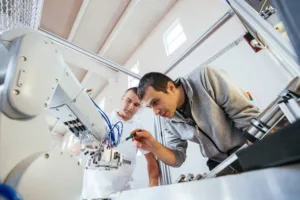
Traditionally, impact factors (IF), determined using a journal’s average citations over the previous two years, are regarded as the gold standard for assessing research quality. However, there are considerable restrictions.
Journal bias: high-impact journals receive more citations, regardless of content, which inflates their scores. Smaller, specialist publications containing significant research frequently suffer.
Field disparity: distinct professions have intrinsically distinct citation methods, making meaningful comparisons across disciplines difficult.
Short-term focus: IF emphasises recent citations while overlooking long-term influence and innovative research that takes longer to achieve attention.
Author bias: well-known writers receive more citations, thereby overshadowing equally good contributions from lesser-known scholars.
These constraints have fueled calls for new evaluation methodologies that provide a more detailed view of research effects. This is where engineering AI research comes in, providing novel tools and methodologies to overcome the constraints of existing measurements.
Uncovering the Hidden Dimensions of Engineering AI
Impact
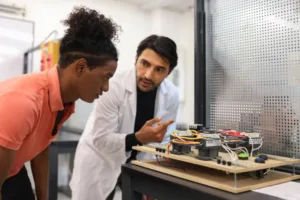
Machine learning, natural language processing, and network analysis are transforming the way we quantify research impact. Here are some important ways in which engineering AI research is demystifying impact factors:
1. Identifying Emerging Areas and Trends: Artificial intelligence algorithms can examine massive volumes of research data to discover emerging areas and research trends before they become widely adopted. This allows researchers to better focus resources and solve significant societal concerns sooner.
2. Going Beyond Citations: Artificial intelligence can assess the content of research publications, including technique, innovation, and prospective applications, to uncover meaningful contributions that may not be reflected in citation counts. This provides a more comprehensive assessment of research quality and impact.
3. Uncovering Hidden Links: AI-powered network analysis can discover previously unknown links between researchers, institutions, and ideas, shedding light on collaboration networks and the flow of knowledge across scientific communities. This helps us understand the true impact of research beyond individual articles.
4. Predicting Future Influence: Machine learning models can use previous citation data to forecast the influence of academic papers. This allows funding agencies and institutions to emphasise research that has the potential to make a major long-term contribution.
5. Customised Metrics for Different Fields: AI may create metrics tailored to different fields, taking into consideration their distinct citation practices and publication patterns. This provides more equitable comparisons and a more accurate assessment of research impact across fields.
Examples of Artificial Intelligence-Powered Tools
Altmetrics: Monitor online mentions, shares, and conversations of research to gain insights into its larger societal influence beyond academic citations.
Scientometric analysis tools: Machine learning is used to evaluate enormous datasets of publications, revealing citation patterns, author networks, and new trends.
Predictive impact models: Analyse past data to forecast the future citation potential of research publications, which can help with funding decisions and resource allocation.
Read More: Unveiling a Quantum AI-Powered Self-Driving Cars
What is the Future of AI-Powered Research Evaluation?
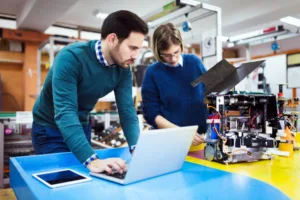
Engineering AI research is not only demystifying impact factors; it is fundamentally changing the way we evaluate research. This transition to more complex and comprehensive measurements has enormous potential.
More equitable evaluation: Moving beyond a single statistic, such as IF, can help academics from various backgrounds and underrepresented fields compete more fairly.
Greater identification of various contributions: AI technologies can discover meaningful research contributions that may not be reflected in standard measurements, allowing for a more comprehensive understanding of research influence.
Improved resource allocation: AI can help funding organisations and institutions allocate money more wisely, focusing on areas with the greatest potential for societal benefit.
Accelerating scientific development: Having a better understanding of research impact can help steer collaborations, uncover knowledge gaps, and accelerate scientific progress.
Conclusion
While obstacles exist, such as potential biases in AI algorithms and ethical concerns about data privacy, the power of engineering AI research to alter research evaluation is evident. By adopting these novel techniques and stepping beyond the constraints of standard measurements, we may build a more equitable, intelligent, and successful system for quantifying scientific impact.